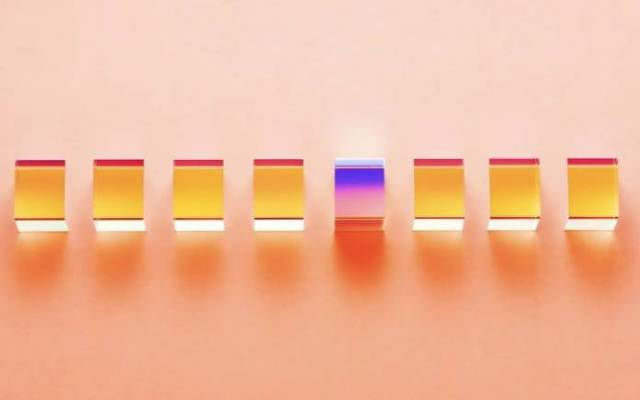
Machine Learning is something not many people will fully understand. It’s vague because the human mind can’t really determine how a computer “reads” and “performs” but simply put, machine learning is the study of statistical models and algorithms that a machine uses. A machine refers to a mobile device or computer. For this purpose, we’re referring to smartphones as we look into how it helps Google Play Store users to see and discover new apps that may be relevant to them.
DeepMind has been helping Google when it comes to AI (artificial intelligence). It is actually a Google company acquired back in 2014, working on AI and related technologies. The search giant has recently detailed the role of its AI in giving recommendations within the Play Store.
Google has been implementing DeepMind’s technology for years now. It has improved how the Play Store discovers apps for the users, choosing more relevant ones. They are more personalized too now.
Play Store’s discovery system has improved over the years. The system has been helping both parties: Google and the users. Actually, the app developers are also benefitting from the system.
With billions of Android users all over the world, it can be difficult to market an app. If you’re a developer, naturally, you’d want to promote your app. App recommendations help but how does machine learning do it exactly? It’s simple: the machine looks into your past user preferences.
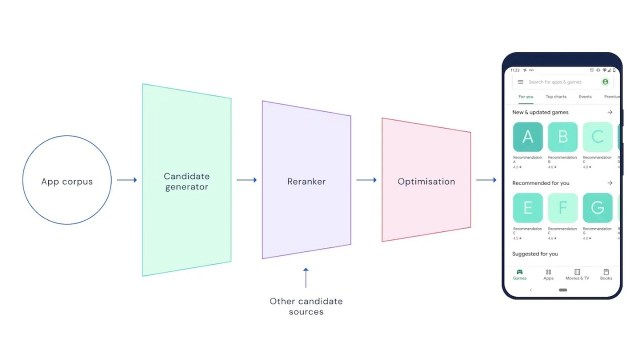
Machine learning works with the Play Store to improve the system. The goal is relevance. Google Play has a recommendation system that has the following: candidate generator, reranker, and a model to optimize multiple objectives. All these work to predict the preferences along multiple dimensions to give candidates to users when needed.
DeepMind deploys a long short-term model (LSTM) to Google Play. This can learn long-term dependencies but there may be a delay at times. It has since been replaced by a Transformer model that has enhanced model performance. Bias can be avoided by looking at importance weighting that includes impression-to-install rate.
Comments